Open Raven at 4: Expanded Coverage, New Automations, and Data Detection & Response
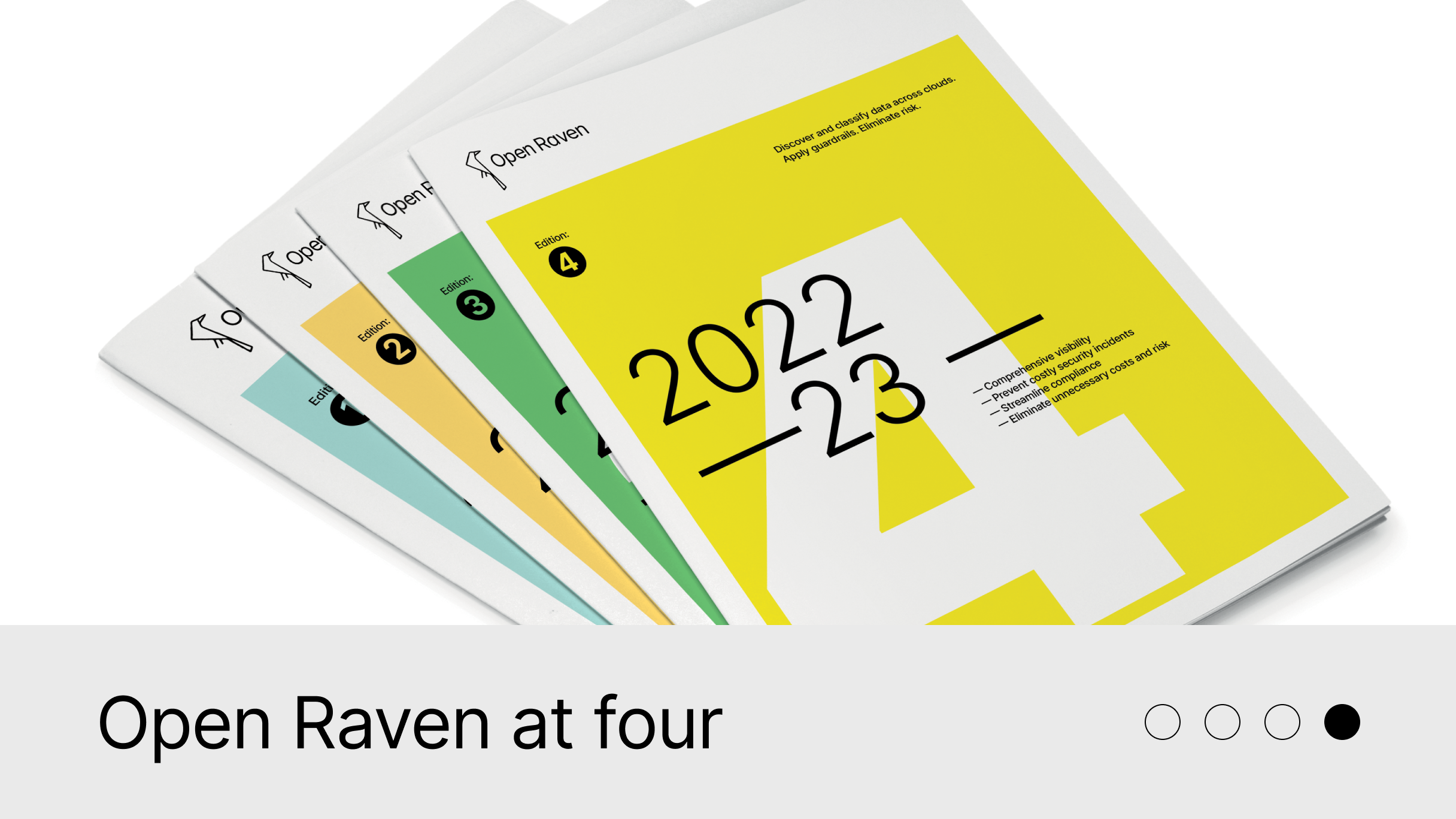
“Oh, so it’s like DLP, right?”
We heard this frequently after starting the company in 2019. The concept of DLP was so deeply entrenched in people’s minds that anything that sounded like data security was immediately stuffed into the metaphorical DLP box. This no doubt speaks to the success of DLP, but also to the lack of other widely deployed data security products.
Fast forward to today. We no longer have to explain what we’re doing, people know Data Security Posture Management (DSPM) by name and its sibling Data Detection & Response (DDR). Gartner put the category in the spotlight at their recent Security & Risk Management Summit, delivering new research, a number of presentations, and making bold predictions about its adoption in upcoming years. Over $1B in investment has been committed to building modern data security solutions since we began in ‘19. The number of companies offering DSPM/DDR has exploded, including a number of legacy companies claiming new DSPM capabilities. It’s exciting, it’s messy, it’s confusing and it’s normal. Perfectly normal for a category in its youth.
Somehow 4 years have passed while we’ve been helping to shape the cloud data security category. That’s a full U.S. presidential term. It’s the usual duration of time one spends in high school. It’s also enough time to see a pandemic, political insurrection, war, and have our bank collapse seemingly overnight. Phew. We consider ourselves incredibly fortunate, blessed even, to be celebrating our 4th birthday.
Announcing a New Board Member
From our earliest days, one of our independent investors distinguished himself as the MVP. His advice, introductions, and the time he invested with us had no match. Instead of losing interest as things progressed, he simply became more valuable until the most obvious thing to do was to offer him a bigger, official role with the company. We’re thrilled to announce that Andrew Peterson accepted and is now a member of the Open Raven Board of Directors.
Andrew brings direct, recent experience navigating a challenging category while scaling up a cybersecurity company from his time at Signal Sciences (ultimately acquired by Fastly). There’s few people in the industry with his combination of broad, strategic vision alongside genuine operational expertise from having built, run and successfully exited a company. Andrew’s partnership with us from day 0 has been invaluable and we’re thrilled to see what we can accomplish together with him as a board member.
DDR, Automations & Expanded Data Source Coverage
We’ve been building against a few major themes this Spring and Summer, namely: 1) expanding data source coverage, 2) making it even easier to automate data security, and 3) adding data detection and response (DDR) capabilities.
Expanding data source coverage
One of the first services we built was DMAP, an ML-based data store fingerprinting capability we used to locate “lifted and shifted” instances of MySQL, CouchDB, etc. on generic compute such as EC2 or GCE. Our rationale was that the operational burden for these services was entirely on the customer, hence the chance of making a mistake was often higher, and cloud tooling frequently could not see these services running. DMAP has frequently identified shadow data that either needs to be removed or pulled into the loving embrace of observability. This Summer, DMAP has received its 4 year tune up and we’ve expanded data inventory and classification to non-native data services such as Postgres and MySQL. Alongside deepening our coverage in AWS, GCP and wrapping up Snowflake support, we now handle the primary data services that drive the data economy.
Automating all the things
No one wants or needs another flood of alerts. CSPM hasn’t been a great experience for many and to the extent that DSPM becomes another work generating system, it falls flat. We originally thought to address this by winnowing down the scope to only the sensitive data in the environment and showing it in a map, asset list and data catalog. This was a great first step and typically has a strong impact. Alerts then focused on data specific issues and used a series of integrations from Slack to Jira and webhooks to complete the loop. This Summer we’re releasing an automations framework that allows for customers to take precise actions based on specific alerts, be it a new data finding or a configuration problem. We do this through a straightforward “if this then that” style of UI. The idea here is that we’ve closed the loop and eliminated toil by allowing for precise actions to be taken without any manual intervention. All the results, none of the sweat.
Data Detect and Response (DDR)
DDR is yet another capability that makes operationalizing data security at scale vastly easier than before. The concept is simple: once you know where all of the sensitive data is (DSPM), you then monitor it for significant events thereafter. What’s a significant event? It could be a dangerous configuration change, signs of ransomware, duplication, attempted deletion, etc. It could also be a significant anomaly from the typical patterns observed over time. DDR is quickly becoming the natural complement to DSPM so that data at scale can be efficiently protected at scale without adding more people or work. You get just the alerts that matter for the most important data you possess and, using the new automation features mentioned above, you can keep response hands-free as well.
Reflections at 4
Milestones are natural times for reflection. So in no particular order, here’s some of what we’ve figured out while trailblazing modern data security.
Free data security is a cheap parachute. When shopping for a parachute, I’m going to assume you wouldn’t head straight to the clearance rack. Instead, you would look for a product you’re willing to trust your life to. The risk is simply too high to lean into that 50% discount. In the same fashion, we found that trust in anything that involves a company’s sensitive data is so important that free solutions are uninteresting. If you’re going to have another company to secure your data, you want assurance that they are directly accountable for its safety. Paying a fair price and having a committed contractual relationship simply makes the most sense. Just like buying a quality parachute recommended by an expert.
Same same but different. At the heart of every data security project is the need for visibility, a desire to prevent leaks, and a requirement to manage compliance risk. After that, the use cases and issues become as unique as the organization itself. Special project with a partner? Set up guardrails to ensure sensitive data stays where it’s expected (e.g., in a VPC, in a specific data store). Need to keep data science teams working safely? Proactively scan and scrub personal data from their workspace. Had an incident and need to know what playbook to run? Scan an account like the hounds of hell are nipping at your heels to see what type of data was in the affected account. And so on. Flexible platforms win the day as a result versus more rigid tooling.
Not much survives a collision with the real world. Similar to the variety in use cases, there is little commonality in the data across organizations. The type of objects will depend somewhat on the industry. The data stores that matter vary widely. The data might be compressed, encrypted (where’s the key?), have no header, the wrong header, or be wildly off-spec with respect to its size and formatting. All bets are off and the safest assumption is that it’s a mess. There’s always custom data classes required and we have not even discussed sampling rates. Now compare this to detecting vulnerabilities or malware, both types of products I’ve been building for over 20 years. The set of things that have to be tested to be baseline effective-- and the corpus of test data-- is smaller and infinitely more predictable. The use cases are predictable and constrained. Bluntly, it’s easier.
LLM all the things! Nope. Artificial intelligence has a clear role in data classification. Time-honored regex-based data classification has clear limits to how effective it can be by itself. Nonetheless, it’s fast, inexpensive and flexible. We’ve long since been using ML to derive highly effective patterns and using ML to verify results. Enter LLMs and widespread use of NLP transformers. Does this truly change the game for data classification for security? Not really. Security and cloud teams simply can’t or won’t be able to use the time and resources to train across all of an organization’s data. And why would you if you can get the same results with equally effective, easier, and less expensive techniques? We believe a complete approach to data classification will require AI, but the exact technique used, from NLP transformers to ML or regex patterns with keyword adjacency, should depend on exactly what’s needed. This is fundamentally about choosing the right tool for the job.